The Rapid Transformation in Recruitment with AI and Machine Learning
The recruitment industry is undergoing a profound transformation, driven by advancements in artificial intelligence (AI) and machine learning (ML). Traditional recruitment methods, often labor-intensive and limited in scope, are being revolutionized by technology that enables hiring teams to work faster and more accurately. By leveraging data-driven insights, AI and ML can streamline sourcing, screening, and engaging with potential candidates in ways that were previously unimaginable. Today, companies are increasingly turning to AI-powered solutions to keep up with competitive hiring demands and make better talent decisions.
The Importance of AI and Machine Learning in Today’s Hiring Landscape
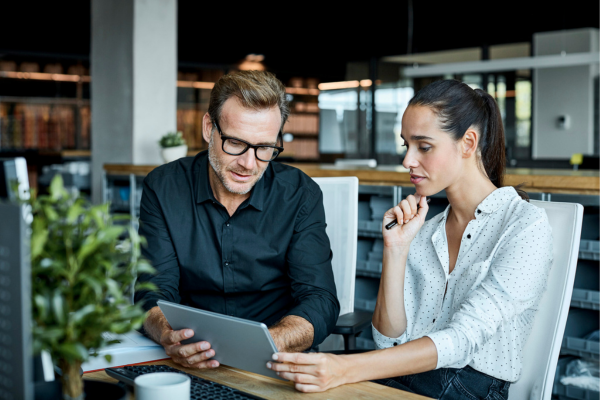
In an era marked by rapid job market changes and evolving skill requirements, AI and machine learning in recruiting have become essential tools for staying competitive. These technologies address critical challenges such as high-volume sourcing, applicant tracking, and candidate experience improvement, making them indispensable for modern recruiters. Machine learning algorithms help recruiters efficiently identify top candidates, ensuring that qualified applicants are not overlooked in the process. As companies continue to face talent shortages in key fields, AI and ML provide solutions that increase hiring quality and reduce time-to-hire, ultimately contributing to stronger, more agile teams.
Overview of Benefits: A Streamlined, Data-Driven Recruitment Process
AI and ML in recruiting offer a suite of benefits designed to refine every stage of the hiring process. From rapid candidate sourcing to automated resume screening and personalized outreach, these technologies reduce administrative tasks, allowing recruiters to focus on strategic decision-making. Additionally, AI and ML mitigate unconscious bias, promoting a more equitable hiring process by focusing solely on skills, qualifications, and relevant experience. By improving recruitment accuracy, reducing hiring timelines, and enhancing the candidate experience, AI and ML serve as powerful tools for modern recruitment.
The Role of AI and Machine Learning in Modern Recruitment
How AI and Machine Learning are Integrated into Recruitment Processes
The integration of machine learning in recruiting is reshaping how companies approach hiring by automating repetitive tasks and providing insights that drive better decision-making. AI-powered systems can analyze vast amounts of candidate data from various sources—such as job boards, social media, and company databases—at a speed and scale that would be impossible for humans to achieve. For example, machine learning algorithms can sort through millions of profiles to identify candidates who best match a job’s requirements, highlighting those with the skills, experience, and cultural fit needed for the role.
Empowering Recruiters with Efficiency and Precision
Machine learning in recruiting is enabling hiring teams to operate with an unprecedented level of efficiency. Instead of manually sifting through resumes, recruiters can use AI-driven tools to automate the initial screening process, allowing them to focus on high-value tasks, like interviewing and candidate engagement. These tools also help ensure that no suitable candidate is overlooked by ranking applicants based on relevant data points, which can include past performance metrics, behavioral assessments, and more. Through this data-driven approach, machine learning supports recruiters in making well-informed hiring decisions that increase the likelihood of long-term success and fit.
By making recruitment faster, more accurate, and less biased, machine learning and AI have established themselves as critical components of modern talent acquisition strategies. This introduction to AI and machine learning in recruitment underscores their significance in today’s competitive landscape and sets the stage for deeper insights into their specific applications in hiring.
Streamlining Candidate Sourcing with AI-Powered Tools
How Machine Learning in Recruiting Scans Databases and Job Boards for Top Talent
One of the standout benefits of using machine learning in recruiting is the capability to process vast amounts of data from multiple sources, including job boards, databases, and social media platforms. Traditional sourcing methods often require extensive time and resources to identify potential candidates across platforms. In contrast, AI-powered recruiting tools can scan these sources within seconds, delivering a curated list of candidates who closely match the qualifications for a given role. This rapid data processing allows recruiters to maintain a steady flow of qualified candidates and reduces the time spent manually sifting through profiles.
By integrating machine learning, these tools continually refine their search and analysis capabilities, learning from previous successful hires to identify traits, skills, and patterns in profiles. This means that as more candidate data is processed, the algorithms become increasingly adept at pinpointing high-quality matches, streamlining the candidate sourcing process even further.
AI’s Predictive Power: Assessing Candidate Fit with Precision
Another significant advantage of machine learning in recruiting is its ability to predict candidate fit with remarkable accuracy. Beyond simple keyword matching, machine learning algorithms assess a candidate’s skills, experience, and even behavioral traits to evaluate their alignment with both the job and company culture. These predictive capabilities extend to analyzing resume and profile data to gauge not only the qualifications but also the potential for long-term success within a particular organization.
For example, machine learning algorithms can evaluate subtle patterns in a candidate’s work history, project types, and soft skills. They analyze these insights against data on current and past employees to recommend candidates who would likely excel within the company’s environment. This approach helps organizations reduce turnover rates and create a better cultural fit, ultimately leading to higher employee satisfaction and retention.
Automating Resume Screening and Candidate Shortlisting
Enhancing Resume Screening with Machine Learning Algorithms
One of the most time-consuming aspects of recruitment is the initial resume screening, where recruiters manually review qualifications, skills, and experience for each candidate. Machine learning in recruiting transforms this process by automating resume screening through intelligent algorithms. These algorithms are designed to identify key qualifications, relevant experience, and specific skills outlined in job descriptions, allowing recruiters to focus on high-quality candidates without the need to manually review every application.
Machine learning algorithms can be customized to screen for particular keywords, credentials, or required certifications, ensuring that only candidates who meet these criteria advance in the hiring process. As a result, recruiters save significant time and resources, enabling them to prioritize candidates with the most potential for success.
AI-Powered Applicant Tracking Systems: Ranking and Sorting Candidates for Efficiency
Applicant Tracking Systems (ATS) powered by AI are essential tools in modern recruiting, especially for companies managing high application volumes. These systems use machine learning to rank and sort candidates based on data-driven criteria, prioritizing those who best align with the role’s requirements. This structured ranking system reduces the likelihood of qualified candidates being overlooked, as the algorithm evaluates each applicant objectively based on their skills and experience.
Additionally, AI-powered ATS can adapt over time, learning from previous successful hires to refine the criteria for future searches. By leveraging machine learning, these systems continuously improve their accuracy, ensuring that the most suitable candidates are recommended for review. This advanced sorting process not only saves recruiters valuable time but also contributes to more consistent and equitable hiring outcomes.
Using AI-Powered Chatbots for Real-Time Candidate Engagement
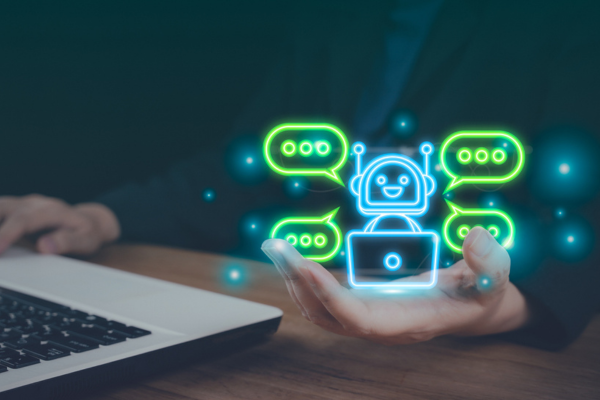
One of the most effective applications of machine learning in recruiting is the use of AI-powered chatbots to enhance the candidate experience. These chatbots interact with candidates in real-time, offering prompt responses to frequently asked questions, guiding applicants through each step of the application process, and providing immediate support. Chatbots can answer inquiries related to job descriptions, application status, company culture, and more, creating a seamless and responsive experience for candidates who may have questions or need additional guidance.
Machine learning enhances these chatbots by continuously improving their responses based on past interactions, ensuring that the information provided is both accurate and relevant. By offering real-time support, chatbots alleviate many common frustrations that candidates face, such as delays in communication or lack of clarity about next steps, fostering a more positive impression of the hiring company.
Sustaining Candidate Engagement and Improving Overall Experience
Chatbots powered by machine learning in recruiting go beyond answering basic questions—they play an essential role in maintaining candidate engagement throughout the application process. This ongoing engagement keeps candidates informed and connected, reducing the likelihood of drop-offs due to delays or unclear communication. By automating these interactions, recruiters have more time to focus on high-value tasks, such as interviewing and relationship building, while candidates receive a responsive and supportive experience.
Furthermore, AI-driven chatbots help tailor the recruitment process to each individual by adjusting their interactions based on specific candidate needs and behaviors. For instance, if a candidate shows interest in a particular role or skill area, the chatbot can share relevant resources, such as blog posts or videos, that help candidates better understand the opportunity and company culture. These customized interactions can significantly enhance the overall candidate experience, building goodwill and fostering stronger interest in the company.
Reducing Bias in the Hiring Process with Machine Learning in Recruiting
Eliminating Unconscious Bias with AI and Machine Learning
One of the most transformative benefits of using machine learning in recruiting is its potential to reduce unconscious bias in the hiring process. Traditionally, human biases—whether intentional or not—can influence recruitment decisions based on factors unrelated to a candidate’s skills or qualifications, such as name, age, gender, or ethnicity. Machine learning algorithms, however, are designed to focus solely on objective data points, such as relevant experience, certifications, and professional skills, making the evaluation process more equitable.
When properly implemented, machine learning algorithms assess candidates based solely on qualifications and relevant data, bypassing subjective factors that might otherwise influence the decision-making process. By relying on algorithms that evaluate skills and qualifications objectively, companies can foster a more inclusive hiring process, ultimately promoting diversity within their workforce.
Promoting Diversity and Inclusion Through AI-Driven Algorithms
AI-driven algorithms are not only designed to eliminate bias but also actively promote diversity and inclusion in recruitment. Some machine learning models are specifically programmed to detect and counteract patterns of bias, ensuring that all candidates receive a fair evaluation. For example, machine learning algorithms can analyze hiring patterns within an organization to identify any existing biases and make adjustments that prioritize diversity in candidate sourcing and evaluation.
By analyzing candidate pools and hiring trends, these algorithms can detect disparities, such as underrepresentation of certain demographics, and adjust the sourcing process to seek out candidates from diverse backgrounds. This proactive approach helps organizations build a more inclusive workforce, creating a culture that values different perspectives and experiences. Through machine learning in recruiting, companies can take significant strides toward equitable hiring practices, setting a standard for fairness and inclusivity in talent acquisition.
Predictive Analytics for Better Talent Matches
Using Machine Learning to Predict Candidate Success
One of the most impactful applications of machine learning in recruiting is predictive analytics, a tool that helps companies make data-driven decisions when assessing talent. Machine learning algorithms analyze historical data from previous hires, including performance metrics, professional backgrounds, and cultural fit, to create profiles of high-performing employees. By examining patterns in this data, machine learning can predict which candidates are most likely to succeed and thrive within the organization.
This predictive approach enables recruiters to go beyond resumes and basic qualifications, considering more nuanced factors that indicate long-term potential. For example, an algorithm might highlight candidates with specific skill combinations, career trajectories, or experiences that align with the characteristics of successful employees. Predictive analytics not only reduces guesswork but also allows recruiters to make more accurate and informed decisions in their candidate selection.
Forecasting Long-Term Success and Reducing Turnover
Predictive analytics also plays a crucial role in identifying candidates likely to excel and remain with the company for extended periods. By evaluating attributes like adaptability, problem-solving abilities, and alignment with company values, machine learning can forecast retention rates and help recruiters select candidates who are not only qualified but are a better fit for the company culture. This insight reduces turnover rates, improving the overall quality of hires and promoting workforce stability.
With machine learning in recruiting, companies can focus on building teams that align with their long-term goals. This proactive approach to hiring saves time, resources, and costs associated with high turnover, ultimately contributing to stronger team cohesion and organizational success.
AI in Interview Scheduling and Talent Assessment
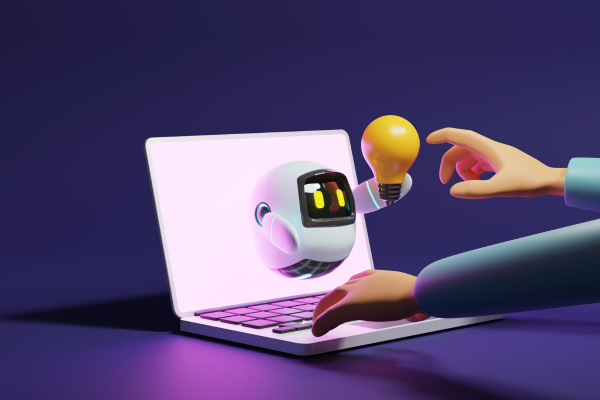
Streamlining Interview Scheduling with AI-Powered Tools
One of the administrative challenges in recruiting is coordinating interview schedules with candidates. Machine learning in recruiting simplifies this process by enabling AI-powered scheduling tools that allow candidates to select available interview slots, eliminating the need for back-and-forth communication. This automated scheduling process ensures that interviews are booked efficiently, reducing wait times and improving the candidate experience.
By automating interview scheduling, recruiters can focus on more strategic aspects of hiring, knowing that candidates are seamlessly progressing through the process. Additionally, machine learning tools can adapt to scheduling conflicts by suggesting alternative slots, making the process even more user-friendly for candidates and recruiters alike.
Leveraging AI for Talent Assessment in Video Interviews
Machine learning also enhances talent assessment through video interview tools that go beyond traditional evaluations. These AI-driven platforms analyze nonverbal cues, such as facial expressions, tone, and language, to provide recruiters with deeper insights into a candidate’s personality and soft skills. For example, machine learning algorithms can detect indicators of confidence, empathy, and problem-solving abilities, helping recruiters make more well-rounded assessments.
This use of machine learning in recruiting enables a more holistic evaluation of candidates, as it captures traits that may not be apparent from resumes alone. By combining traditional assessments with AI-driven insights, recruiters can make better-informed decisions, selecting candidates who not only meet technical requirements but also align with the company’s values and culture.
Leveraging AI for Personalized Candidate Outreach
Crafting Targeted Outreach Messages with AI
Machine learning in recruiting also supports personalized candidate outreach by analyzing data on candidate preferences, behaviors, and engagement histories. With these insights, AI-driven tools can craft tailored outreach messages that resonate with top candidates, improving response rates and fostering connections with passive talent. For example, AI might generate messages that highlight specific job aspects or company values that align with a candidate’s interests, increasing the chances of a positive response.
Personalized outreach helps recruiters engage candidates more effectively, especially those who may not be actively seeking new opportunities. This targeted approach to communication builds relationships and keeps passive candidates connected to the organization, opening the door for future hiring opportunities.
Optimizing Engagement with AI-Driven Recommendations
Machine learning algorithms can also recommend the best communication channels and optimal times to engage with candidates based on past interactions and candidate preferences. For instance, AI may suggest email or LinkedIn outreach for certain candidates or recommend engagement during specific times of the day when candidates are most likely to respond. These insights allow recruiters to connect with talent more effectively and at the right moments, making each interaction more meaningful and improving the overall recruitment process.
By leveraging machine learning in recruiting, companies can develop more precise and engaging outreach strategies, ensuring that top candidates are not only identified but actively engaged. This approach enhances the candidate experience, strengthens employer branding, and ultimately leads to higher-quality hires.
How Machine Learning in Recruiting Evolves with New Data
A unique advantage of machine learning in recruiting is its capacity for continuous learning. As new data from job markets, hiring patterns, and candidate feedback becomes available, machine learning models evolve, improving the accuracy and effectiveness of their recommendations. These models learn from each interaction—whether it’s candidate engagement data, hiring outcomes, or changing skill requirements—refining their algorithms to optimize talent sourcing strategies over time.
This process allows recruiting tools to become more precise and relevant as they adapt to emerging trends, such as increased demand for digital skills or shifts in candidate preferences. This continuous learning loop enhances both the quality of hire and the recruiter’s ability to meet future talent needs efficiently, ensuring that recruitment efforts remain aligned with the latest industry requirements.
Adapting to Market Conditions and Emerging Skills with AI
Machine learning systems in recruiting also adjust dynamically to changes in the job market, such as economic shifts, skill shortages, and emerging industry demands. For example, during periods of economic growth, recruitment models may emphasize high-demand roles and hard-to-find skills. Alternatively, in economic downturns, these systems can prioritize efficiency and cost-effectiveness, helping companies optimize hiring budgets.
By tracking real-time labor trends and analyzing evolving job requirements, AI systems remain responsive and flexible, ready to help recruiters find candidates with in-demand skills. This adaptability equips recruiting teams to make strategic hiring decisions based on the latest information, positioning the organization for success.
Measuring the Success of AI-Driven Recruitment
Key Performance Indicators (KPIs) to Track AI Recruitment Effectiveness
To evaluate the impact of machine learning in recruiting, companies can monitor specific KPIs that highlight the effectiveness of AI-driven strategies. Time-to-hire and cost-per-hire are two critical metrics; reducing these can signify that AI is streamlining processes and cutting costs. Additionally, candidate satisfaction—often measured through post-hire surveys—provides insight into the candidate experience and can indicate if AI-driven interactions, such as chatbot responses, positively influence candidates.
Other KPIs, like quality of hire and retention rate, offer insight into how well AI algorithms are predicting candidate success and cultural fit. By regularly tracking these metrics, companies can measure the impact of AI on recruitment and adjust their strategies to maximize efficiency and effectiveness.
Evaluating ROI from Machine Learning in Recruiting
Beyond KPIs, assessing the return on investment (ROI) of machine learning in recruiting is essential for understanding its long-term value. ROI metrics can include savings from reduced recruiter workloads, improved hire quality, and decreased turnover due to better talent matching. By comparing hiring expenses and productivity gains, companies can determine whether machine learning tools are generating measurable returns.
For example, if AI recruiting tools consistently lead to faster hiring and lower turnover, the ROI justifies the initial investment in these technologies. Regular ROI evaluations help recruiters refine their machine learning strategies, ensuring they continue to optimize hiring processes effectively.
Incorporating AI and machine learning into recruitment offers companies a powerful way to streamline hiring, reduce bias, and enhance candidate experiences, making it an essential strategy in today’s competitive job market. For businesses facing high-volume hiring or specialized skill shortages, these tools provide the data-driven insights needed for more efficient and effective talent acquisition. To explore how AI and machine learning can elevate your hiring process, request a demo from GoToro and discover firsthand the transformative impact of AI-driven recruitment solutions.